3rd GNNet Workshop
Graph Neural Networking Workshop
Co-located with ACM CoNEXT 2024
December 9, 2024
We are glad to announce the “3rd Graph Neural Networking Workshop 2024”, which is organized as part of “ACM CoNEXT 2024” to be held in Los Ángeles. All accepted papers will be included in the conference proceedings and be made available in the ACM Digital Library.
Motivation
Workshop goal
The goal of GNNet is to leverage graph data representations and modern GNN technology to advance the application of AI/ML in networking. GNNet provides the first dedicated venue to present and discuss the latest advancements on GNNs and general AI/ML on graphs applied to networking problems. GNNet will bring together leaders from academia and industry to showcase recent methodological advances of GNNs and their application to networking problems, covering a wide range of applications and practical challenges for large-scale training and deployment.
We expect GNNet would serve as the meeting point for the growing community on this fascinating domain, which has currently not a specific forum for sharing and discussion.
The GNNet workshop seeks for contributions in the field of GNNs and graph-based learing applied to data communication networking problems, including the analysis of on-line and off-line massive datasets, network traffic traces, topological data, cybersecurity, performance measurements, and more. GNNet also encourages novel and out-of-the-box approaches and use cases related to the application of GNNs in networking. The workshop will allow researchers and practitioners to discuss the open issues related to the application of GNNs and graph-based learning to networking problems and to share new ideas and techniques for big data analysis and AI/ML in data communication networks.
This edition welcomes not only applications of GNN models to problems in communication systems, but also the application of novel ML solutions based on graph representations, such as Transformers, Geometric Deep Learning, Topological Deep Learning, or any other graph-based ML methods
Keynotes
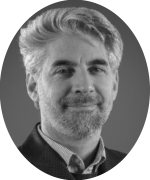
Marco Mellia
Marco Mellia is a full professor at Politecnico di Torino, Italy, where he is the coordinator of the SmartData@PoliTO center on Big Data, Machine Learning and Data Science. His research interests are in the area of Internet monitoring, users’ characterisation, cyber security, and big data analytics applied to different areas. He has co-authored over 250 papers published in international journals and presented in leading conferences. He won the IRTF ANR Prize at IETF-88, and best paper award at IEEE P2P’12, ACM CoNEXT’13, IEEE ICDCS’15. He is Fellow of IEEE and Editor in Chief of the Proceedings of the ACM on Networking.
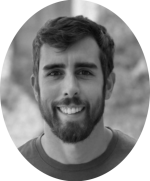
Guillermo Bernárdez
Guillermo Bernárdez is a Postdoctoral Researcher at UC Santa Barbara, where he works in the Geometric Intelligence lab lead by Prof. Nina Miolane. He holds a PhD in the area of Machine Learning applied to Computer Networks at Universitat Politècnica de Catalunya, and a MSc degree in Artificial Intellicence from the same university. Previously he received his BSc degrees in Mathematics and Physics from Universitat de Barcelona. During 2023, he was a visiting researcher at the University of Cambridge (UK) under the supervision of Prof. Pietro Lio, where he started working on the fields of Topological and Geometric Deep Learning —currently his main research topics.
Topics of Interest
We encourage both mature and positioning submissions describing systems, platforms, algorithms and applications addressing all facets of the application of GNNs and Machine learning on graphs to the analysis of data communication networks. We are particularly interested in disruptive and novel ideas that permit to unleash the power of GNNs in the networking domain. The following is a non-exhaustive list of topics:
- GNNs and graph-based learing in networking applications
- Representation Learning on networking-related graphs
- Application of GNNs to network and service management
- Application of GNNs to network security and anomaly detection
- Application of GNNs to detection of malware, botnets, intrusions, phishing, and abuse detection
- Adversarial learning for GNN-driven networking applications
- GNNs for data generation and digital twining in networking
- Temporal and dynamic GNNs in networking
- Graph-based analytics for visualization of complex networking applications
- Libraries, benchmarks, and datasets for GNN-based networking applications
- Scalability of GNNs for networking applications
- Explainability, fairness, accountability, transparency, and privacy issues in GNN-based networking
- Challenges, pitfalls, and negative results in applying GNNs to networking applications
- Transformers, Geometric Deep Learning and Topological Deep Learning applied to computer networks
Submission Instructions
Submissions must be original, unpublished work, and not under consideration at another conference or journal. Submitted papers must be at most six (6) pages long, including all figures, tables, references, and appendices in two-column 10pt ACM format. Papers must include authors names and affiliations for single-blind peer reviewing by the PC. Authors of accepted papers are expected to present their papers at the workshop.
All accepted papers will be included in the conference proceedings and be made available in the ACM Digital Library.
Please submit your papers at https://conext-gnnet2024.hotcrp.com/
Important dates
- Paper submission deadline:
July 15, 2024July 31, 2024 (hard deadline) - Paper acceptance notifications:
August 31, 2024September 10, 2024 (extended) - Camera ready due:
September 23, 2024September 30, 2024 (extended) - Workshop date: December 9, 2024
Please note that these dates are tentative and may still change slightly in the next few weeks. Please stay tuned for further updates.
Workshop Chairs
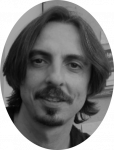
Pere Barlet-Ros
BNN-UPC, Spain
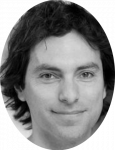
Pedro Casas
AIT, Austria
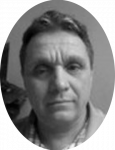
Franco Scarselli
University of Siena, Italy
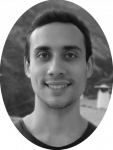
José Suárez-Varela
Telefónica Research
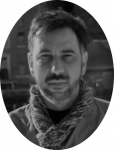
Albert Cabellos
BNN-UPC, Spain
Program Committee
- Paul Almasan, Telefonica Research, Spain
- Zied Ben Houidi, Huawei Technologies Co. Ltd, France
- Lilian Berton, University of Sao Paulo, Brazil
- Ismael Castell, Universitat Politecnica de Catalunya, Spain
- Lluís Fàbrega, Universitat de Girona, Spain
- Jérôme François, INRIA, France
- Fabien Geyer, Technical University of Munich, Germany
- Matthias Herlich, Salzburg Research, Austria
- Brigitte Jaumard, Concordia University, Canada
- Mehrdad Kiamari, University of Southern California, USA
- Federico Larroca, Universidad de la República, Uruguay
- Alina Lazar, Youngstown State University, USA
- Jens Myrup Pedersen, Aalborg University, Denmark
- Christoph Neumann, Broadpeak, France
- Xi Peng, Huawei Technologies Co. Ltd, Hong Kong, China
- Dario Rossi, Huawei Technologies Co. Ltd., France
- Krzysztof Rusek, AGH University of Science and Technology, Poland
- Santiago Segarra, Rice University, USA
- Pavlos Sermpezis, Aristotle University of Thessaloniki, Greece
- Stefano Traverso, Ermes Cyber Security SRL, Italy
- Zhi-Li Zhang, University of Minnesota – Twin Cities, USA